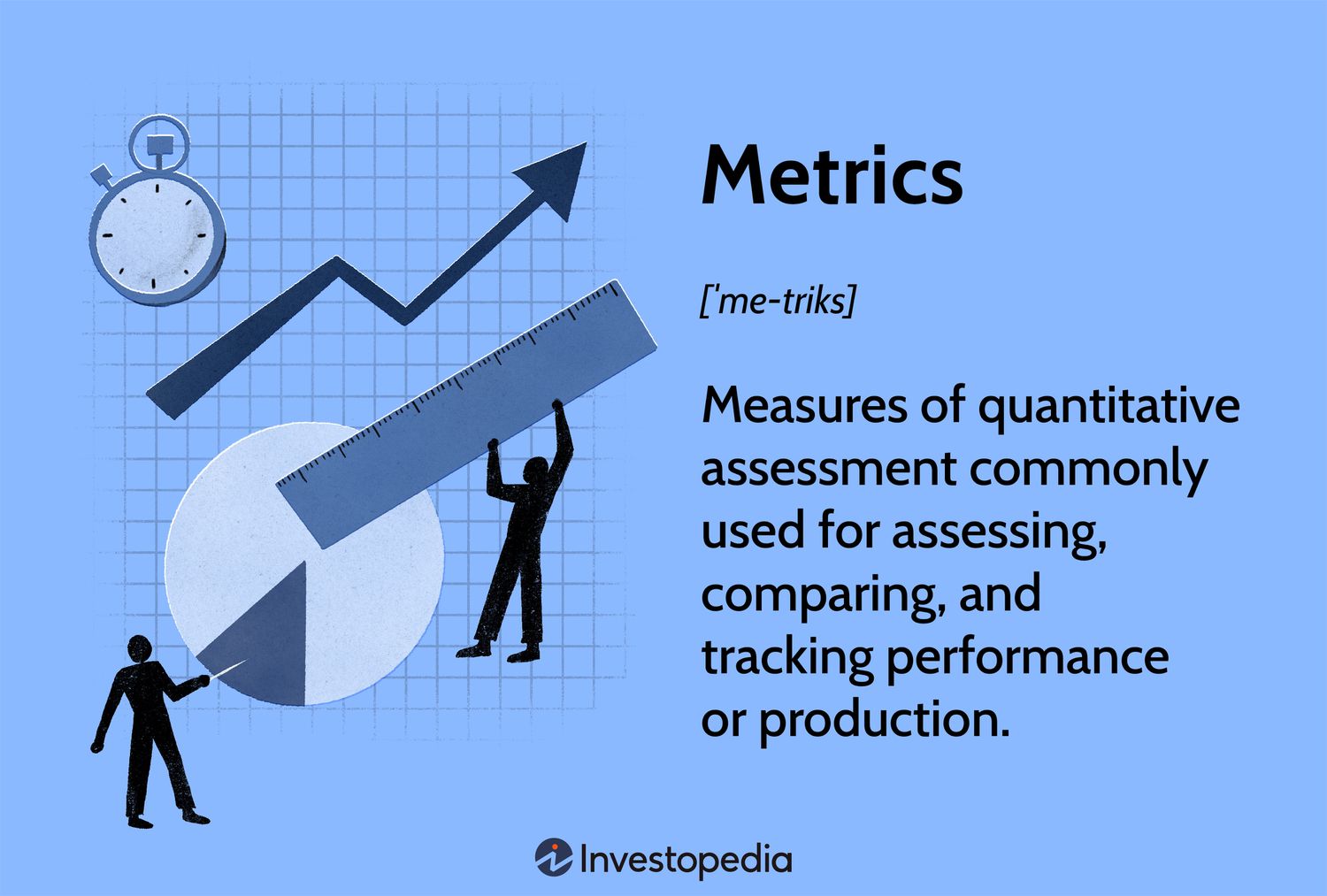
Effective financial metrics analysis needs avoiding common pitfalls. Many businesses find hard to interpret financial data. This leads to costly errors affecting performance. We will explore major mistakes in financial metrics analysis to help make evaluations accurate and actionable.
First, data quality is important. You need clear definitions of key metrics that matter. Focus on metrics that reflect success. Also, using technology can enhance analysis. Analyzing historical data is vital for trend insights.
By knowing and avoiding these mistakes, you can navigate complexities in financial analysis. This leads to better strategic decisions for business. Dive in and elevate your financial metrics analysis to foster growth and succeed.
Common Mistakes in Financial Metrics Analysis
Financial metrics analysis play a vital role in decision making for businesses. However, many pitfalls can lead to incorrect conclusions. A major mistake involves not understanding the context of financial data. Comparing revenue metrics across different sectors without considering their operational differences leads to incorrect judgments. This lack of context causes misguided business strategies not in line with reality.
Another common error is the neglect of relationships among various financial metrics. When metrics are isolated, it creates oversimplified conclusions. For example, focusing only on revenue growth while ignoring profitability can hide significant inefficiencies. Such oversights pose risks to future growth and financial health.
Decision-makers often prioritize vanity metrics that provide no actionable insight. Metrics like total user count may look good, yet they don’t always indicate engagement or profitability. Organizations might waste resources fixing superficial metrics instead of focusing on critical areas that foster long-term success.
Reliance on outdated financial data is another big mistake in financial metrics analysis. Companies that don’t analyze past data for trends or seasonal shifts often misinterpret their current financial state. Historical analysis offers crucial insights for forecasting challenges and strategic planning.
Furthermore, unclear presentation of findings can obstruct decision making. When analyses lack clarity, even the most precise data create confusion among stakeholders. Proper reporting and interpretation of financial metrics are key for all parties to grasp the data’s implications.
These errors emphasize the importance of thorough financial metrics analysis and precise reporting in driving effective business strategies. As we move to the next section, we’ll see that ignoring data quality is one of the biggest mistakes companies must avoid for reliable analysis.
Overlooking Data Quality
A common mistake in financial metrics analysis is overlooking data quality. Bad data can harm the accuracy of analyses. This leads to poor decisions. In finance, good data forms the groundwork for all evaluations. If the base is wrong, every metric afterward is questionable.
Using precise data is essential for reliable financial metrics analysis. Research indicates that around 30 percent of firms encounter losses due to data quality. These losses can stem from financial errors or missed opportunities. They can also cause threats to a firm’s reputation. Regulatory penalties can add to these risks as companies with poor data handling might face fines.
To maintain quality data, firms should adopt several best practices. Firstly, a strong data governance framework is key. This should contain specific guidelines for entering data and for validation processes. Regular data audits can help catch errors early. Also, promoting a culture where staff values data integrity improves handling. Using automated tools for data cleansing can help keep quality checks ongoing. This streamlining helps ensure the data quality remains strong across various systems.
In conclusion, neglecting data quality can ruin financial metrics analysis, impacting decision-making. Moving to the next area, on the lack of clear metrics definition, it’s vital to remember that any analysis depends on high-quality data. If the definitions are hazy, then the analysis might end up being useless or damaging.
Failing to Define Key Metrics Clearly
In financial metrics analysis, it’s essential that key performance indicators must be clear to track business effectively. Vague metrics lead to confusion which can hurt proper decision-making. Stakeholders who are misaligned on metric meanings can distort data analysis.
An example is when a company has a loose definition for customer satisfaction. This allows subjective interpretations to arise. If satisfaction is not well defined, it can cause inconsistencies in how data gets collected, which can mislead the level of actual satisfaction. Poorly defined metrics complicate gauging financial performance.
In addition, unclear definitions may cause wrong resource allocations and strategies. One analysis shows that 40% of companies struggle with clear KPI definitions. This can lead teams to focus on projects not matching actual financial goals, which can hurt performance.
To avoid these issues, organizations needs a clear framework for defining and sharing key metrics. Ensuring stakeholders understand each KPI, its calculations, and its importance to financial strategy is vital. A consistent terminology and standard procedures for metrics analysis can improve data integrity and strategic decision-making.
Furthermore, as we proceed, organizations should be cautious of vanity metrics. These metrics can appear impressive but often do not offer real insights into financial performance. Understanding the difference is key in financial metrics analysis.
Relying on Vanity Metrics
In financial metrics analysis, distinguishing meaningful metrics from vanity metrics is vital. Vanity metrics look impressive but do not reflect a business’s actual performance. Examples are user numbers, social media likes, or website visits without context on engagement. These figures can mislead about a company’s success.
Businesses often prioritize vanity metrics and waste resources. Focusing on appealing numbers might cause firms to ignore customer acquisition costs, churn rates, and lifetime customer value. These metrics reveal the actual business performance and financial condition.
Heavy reliance on vanity metrics can lead to poor strategies. A startup might celebrate increased web traffic without checking if it results in leads. Metrics that don’t affect finances can create false confidence and harm growth. It’s vital to know that measurability does not equal meaningfulness.
To avoid pitfalls from vanity metrics, a focus on metrics that indicate financial success is necessary. Create a framework that emphasizes metrics aligned with business strategy and goals. Instead of only tracking website visits, evaluate engagement, bounce rates, and conversion rates for insights about performance.
Furthermore, companies should adopt actionable metrics for better decision-making. For instance, knowing customer retention rates indicates long-term health, yet monitoring satisfaction and net promoter scores can quickly reveal retention problems. This ensures a focus on metrics that influence financial outcomes.
Understanding the value of selecting the right metrics is crucial, but analyzing historical data is also important. Historical performance insights can inform future decisions and help avoid the pull of vanity metrics.
Neglecting Historical Data Analysis
In the field of financial metrics analysis, organizations often make a key mistake. They overlook the insights that historical data offers. Historical data provides vital information for accurate financial forecasting. Analyzing past performance uncovers patterns. This helps in understanding market changes and spotting future challenges. Organizations risk poor choices about financial health without this context.
Historical trends in financial metrics analysis help shape future financial strategies. For instance, when a company sees a regular sales increase during the holidays from years of data, it can prepare wisely. They can adjust resources, manage inventory, and align marketing efforts. This drives operational efficiency and boosts revenue potential during busy seasons.
In financial metrics analysis, it’s crucial to choose relevant historical data points. Important metrics may include sales volumes over time, cash flow statements, profit margins, and expense patterns. Factors like economic situations or shifts in industry rules may also impact historical data interpretation. A broader historical data range helps organizations make better forecasts and financial decisions ahead.
As we discuss technology’s role in metrics analysis, understanding how historical data integrates with analytical tools is key. This combination can enhance predictions and planning in finance.
Using Technology For Enhanced Metrics Analysis
The landscape of financial metrics analysis constantly changes. Using technology greatly improves the accuracy and efficiency of data interpretation. Many software tools help streamline the analysis process, making it easier for businesses to turn raw financial data into actionable insights.
Business intelligence platforms tailored for the car wash industry have many functions. These tools let operators track important performance metrics in real-time. Their dashboards show vital data, making it simpler for leaders to understand performance trends. By using such tools, businesses make their financial metrics analysis real-time and easy to understand.
Automation in financial metrics analysis provides another noteworthy benefit. Automated systems lessen human error risk, maintaining financial data integrity. Repetitive tasks, like data entry, can be automated, saving time. This shift allows financial analysts to engage in deeper analysis. For example, automating sales and expenses reports may enhance reporting efficiency by 30%, freeing up teams for strategic decisions.
Moreover, advanced analytics software can reveal hidden trends and insights not obvious with manual review. These systems often use machine learning algorithms for predicting future trends based on past data, improving forecasting accuracy. Ignoring historical data can result in bad financial choices, but technology supports businesses analyze past data for better future predictions.
In conclusion, technology use in financial metrics analysis not only streamlines processes but improves insight quality from financial data. Choosing suitable business intelligence tools and automation allows organizations to dodge common issues. This improves decision-making, which is crucial in today’s competitive market.
Conclusion
Effective financial metrics analysis crucial for informed decision-making and business success. This article highlighted pitfalls like poor data quality and ignoring clear definitions of key metrics. We discussed the risks of vanity metrics and the importance of historical data. Also, we saw how technology helps enhance metrics analysis.
You know the mistakes now, and it’s time to act. Start by reassessing your current financial metrics analysis methods. Check if your data is trustworthy, and focus on key metrics that show your organization’s performance well. Use these insights to sharpen your analysis and improve financial decisions.
Mastering financial metrics analysis takes time but can benefit your business greatly. Embrace these practices, and you’ll strengthen your position to navigate a complex financial landscape smoothly.
About WashMetrix
WashMetrix is a cutting-edge business intelligence platform tailored for the car wash industry, delivering robust data analytics to enhance financial tracking and operational efficiency.
By centralizing important metrics and providing actionable insights, WashMetrix empowers car wash operators of all sizes to optimize their performance and drive growth. Discover how WashMetrix can transform your car wash business today!
More from this Category
Recent Post
Absolutely amazing dolor sit amet beyond compare